Research > Semantic Web
Topics
Funding
Grant Number: 1R01HL087795-01A1
NATIONAL HEART, LUNG, AND BLOOD INSTITUTE (NHLBI), NATIONAL INSTITUTES OF HEALTH (NIH)
Principal Investigator: Amit Sheth, Kno.e.sis Center, Wright State University
Co-Principal Investigator: Rick Tarleton, Center for Tropical and Emerging Global Diseases (CTEGD), University of Georgia
Co-Principal Investigator: Mark Musen, National Center for Biological Ontologies (NCBO), Stanford University
Co-Principal Investigator: Natasha Noy, National Center for Biological Ontologies (NCBO), Stanford University
Co-Principal Investigator: Prashant Doshi, Large Scale Distributed Information Systems (LSDIS) Lab, University of Georgia
The study of complex biological systems increasingly depends on vast amounts of dynamic information from diverse sources. The scientific analysis of the parasite Trypanosoma cruzi (T.cruzi), the principal causative agent of human Chagas disease, is the driving biological application of this proposal. Approximately 18 million people, predominantly in Latin America, are infected with the T.cruzi parasite. As many as 40 percent of these are predicted eventually to suffer from Chagas disease, which is the leading cause of heart disease and sudden death in middle-aged adults in the region. Research on T. cruzi is therefore an important human disease related effort. It has reached a critical juncture with the quantities of experimental data being generated by labs around the world, due in large part to the publication of the T.cruzi genome in 2005. Although this research has the potential to improve human health significantly, the data being generated exist in independent heterogeneous databases with poor integration and accessibility. The scientific objectives of this research proposal are to develop and deploy a novel ontology-driven semantic problem-solving environment (PSE) for T.cruzi. This is in collaboration with the National Center for Biomedical Ontologies (NCBO) and will leverage its resources to achieve the objectives of this proposal as well as effectively to disseminate results to the broader life science community, including researchers in human pathogens. The PSE allows the dynamic integration of local and public data to answer biological questions at multiple levels of granularity. The PSE will utilize state-of-the-art semantic technologies for effective querying of multiple databases and, just as important, feature an intuitive and comprehensive set of interfaces for usability and easy adoption by biologists. Included in the multimodal datasets will be the genomic data and the associated bioinformatics predictions, functional information from metabolic pathways, experimental data from mass spectrometry and microarray experiments, and textual information from Pubmed. Researchers will be able to use and contribute to a rigorously curated T.cruzi knowledge base that will make it reusable and extensible. The resources developed as part of this proposal will be also useful to researchers in T.cruzi related kinetoplastids, Trypanosoma brucei and Leishmania major (among other pathogenic organisms), which use similar research protocols and face similar informatics challenges.
Project Funding: $1500000
Project Period: 2008 - 2012
Grant Number: N/A
A MICROSOFT SENSORMAP 2007 RFP AWARD
Principal Investigator: Prashant Doshi, Computer Science Department, UGA
In both computer science and information science, ontology is a data model that represents a set of concepts within a domain and the relationships between those concepts. It is used to reason about the objects within that domain. Ontologies are used in artificial intelligence, the semantic web, software engineering and information architecture as a form of knowledge representation about the world or some part of it. As ontologies become the preferred ways for storing data, sensor data providers are likely to develop detailed ontologies for their sensor data descriptions. However, currently envisioned and realized frameworks for publication of sensor data, such as the SenseWeb, do not provide a way to utilize provider-defined ontological representations of the sensor data. Instead, they require the provider to undertake potentially tedious and complex ways of registering their sensor feed data with them. Our research considers the provider-defined data models and automatically identifies and semantically aligns relevant concepts from the provider-defined data models with those of the publisher's data models. In addition, we are investigating methods by which the provider data models may be appropriately merged into the publisher's data models, thereby transforming the, possibly minimal, sensor types of the publisher into richer explicit data. This approach will not only alleviate the burden on the data provider by allowing reuse of existing data representations, it will also reduce the burden on the data publisher by avoiding the need to develop detailed data models of the different sensor types. Furthermore, the richer ontologies that result may be used to facilitate refined queries of the sensor data and new combinations with other existing data feeds. The data of third-party sensor feeds will be obtained and primarily used for evaluation. Part of this study includes a proposal to the University of Georgia's campus transit board to set-up a wireless sensor network for tracking the campus bus shuttles with plans for publishing the sensor data on the SensorMap portal. This research is significant because it represents a major step toward automating the publication of sensor data feeds with minimal human effort involved.
Project Funding: $53864
Project Period: 2007 - 2008
Automated alignment of ontologies
Research is focusing on a new method for mapping ontology schemas that address similar domains. The problem of ontology matching is crucial since we are witnessing a decentralized development and publication of ontological data. The problem of inferring a match between two ontologies is formulated as a maximum likelihood problem, and solved using the technique of expectation-maximization (EM). The research seeks to exploit the structural, lexical and instance similarity between the graphs, and differs from the previous approaches in the way it utilizes them to arrive at, a possibly inexact, match. Inexact matching is the process of finding a best possible match between the two graphs when exact matching is not possible or is computationally difficult. In order to scale the method to large ontologies, the research identifies the computational bottlenecks and adapts the generalized EM by using a memory bounded partitioning scheme. Researchers have developed a framework called Optima that implements the ontology alignment framework and applied it to semantic reconciliation of sensor metadata for publication in Microsoft's SensorMap.
Learn More >>Knowledge enabled querying of biological data
As part of a bigger project, researchers are investigating a knowledge-driven query formulation system called Cuebee, targeted at domain scientists such as biologists. To support OWL ontologies, it has been integrated with an OWL-DL reasoner called Pellet. Pellet enables semantic reasoning over ontologies and datasets and utilizes SPARQL-DL, an extension of the SPARQL query language. Cuebee offers query composition using intuitive drop-down lists and browse-and-select facilities on high level concepts and relations which have been defined for the parasite Trypanosoma cruzi. In collaboration with biologists, researchers are planning to integrate semantic query formulation with Web services such as NCBI's BLAST and multiple datasets.
Learn More >>Collaborators
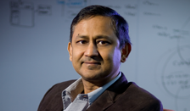
Amit Sheth
LexisNexis Professor of Computer Science Director of the Kno.e.SiS Center Wright State University, USA
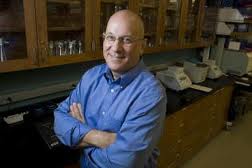
Rick L. Tarleton
Distinguished Research Professor Department of Cellular Biology University of Georgia, USA